Image Annotation: Enhancing AI with Precise Data Labeling
Image Annotation: Enhancing AI with Precise Data Labeling
Blog Article
Introduction:
In the realm of artificial intelligence (AI) and machine learning (ML), image annotation is essential for training models to identify and interpret visual information. This process is integral to various applications, ranging from autonomous vehicles to healthcare diagnostics, as it provides meticulously labeled datasets that enable machines to learn effectively. This article will delve into the concept of image annotation, its various types, applications, and the best practices for ensuring high-quality annotations.
Defining Image Annotation
Image Annotation refers to the technique of tagging images with pertinent metadata to enhance their comprehensibility for machines. This process includes the application of tags, bounding boxes, segmentation masks, or other indicators to objects within an image. The resulting annotated images serve as training datasets for AI and ML models, allowing them to recognize patterns, categorize objects, and make informed decisions based on visual data.
Categories of Image Annotation
There are several categories of image annotation, each tailored to specific needs based on the AI application:
1. Bounding Box Annotation
- This method is utilized in object detection tasks.
- Objects are enclosed within rectangular boxes, facilitating easier recognition by AI.
2. Semantic Segmentation
- This technique assigns a label to each pixel in an image.
- It is particularly beneficial for applications such as medical imaging and self-driving technology.
3. Instance Segmentation
- This approach is akin to semantic segmentation but distinguishes between different instances of the same object category.
4. Keypoint Annotation
- This method identifies specific points on an object, such as facial features or joint locations in human pose estimation.
5. Polygon Annotation
- This technique employs multiple points to accurately outline the shape of an object.
- It is especially useful for objects with irregular shapes, such as road signs or organic forms.
6. 3D Cuboid Annotation
- This technique enhances depth perception in object recognition.
- It is crucial for applications such as robotics and augmented reality.
7. Polyline Annotation
- This method is utilized for lane detection in autonomous vehicles.
- It aids AI models in comprehending road boundaries and markings.
Applications of Image Annotation
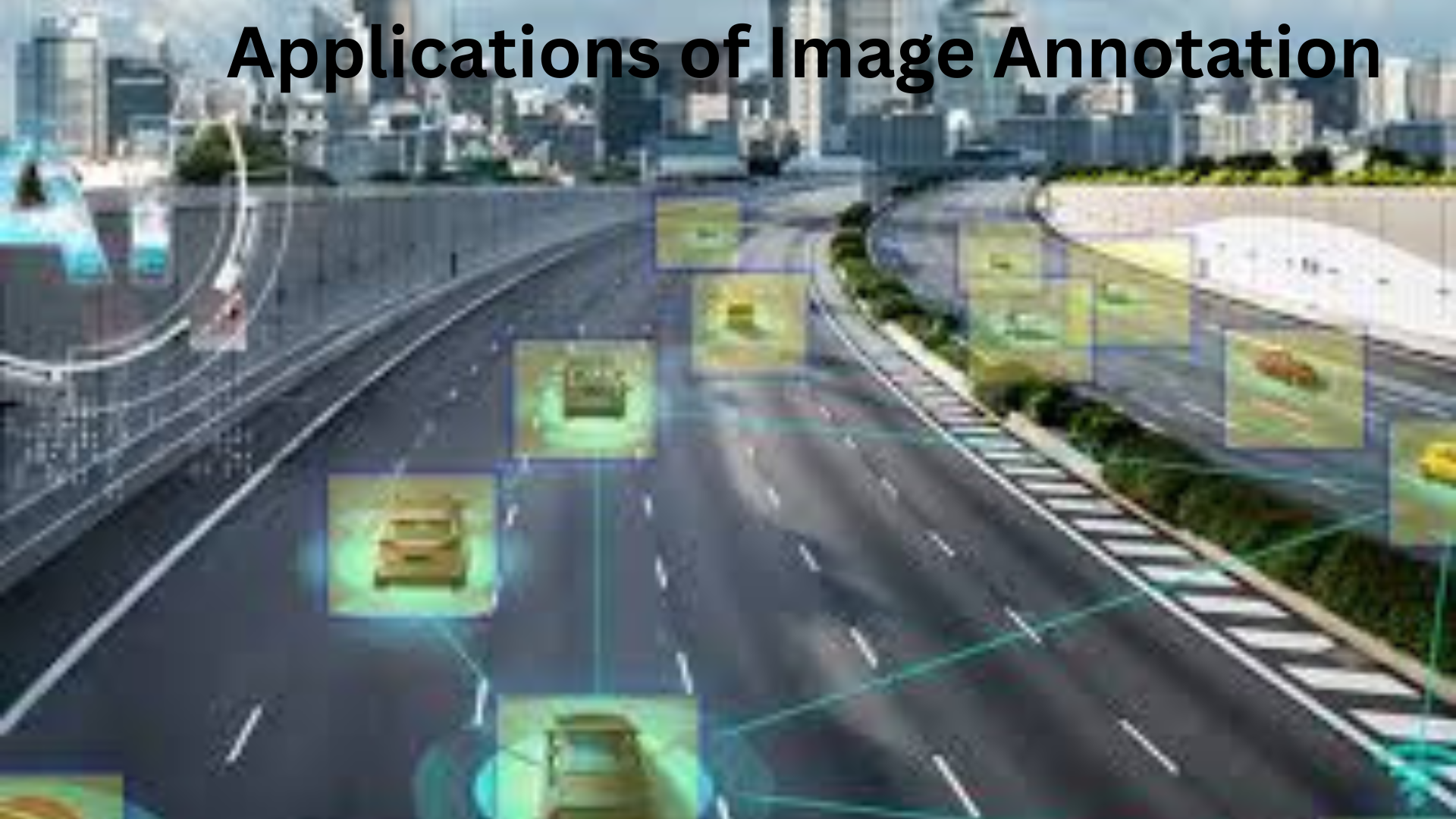
Image annotation finds extensive use across various industries, fostering innovation in multiple domains:
- Autonomous Vehicles – Facilitates the ability of self-driving cars to identify pedestrians, other vehicles, traffic signs, and lane markings.
- Healthcare – Supports the analysis of medical images, including tumor identification in MRIs and X-rays.
- Retail & E-commerce – Enhances visual search capabilities, product recommendations, and automated inventory management.
- Agriculture – Assists in monitoring crops, detecting pests, and implementing precision farming techniques.
- Security & Surveillance – Improves facial recognition systems and the detection of suspicious activities.
Best Practices for Effective Image Annotation
- Consistency in Labeling – Adhere to uniform labeling standards to ensure the accuracy of data.
- Use High-Quality Images – Utilizing clear, high-resolution images enhances AI training outcomes.
- Leverage Automation – Employ AI-assisted annotation tools to expedite the annotation process.
- Human-in-the-Loop Approach – Integrate automated tools with human oversight to achieve greater precision.
- Diverse Data Representation – Ensure that datasets encompass a variety of angles, lighting conditions, and object variations.
Conclusion
Image annotation serves as a fundamental component of AI-powered computer vision applications. As artificial intelligence progresses, the necessity for high-quality annotated datasets is increasing. In sectors such as autonomous driving, healthcare, and retail, accurate image annotation enables machines to enhance their decision-making capabilities, fostering innovation and efficiency throughout various industries.
For exceptional image and video annotation services, consider visiting Globose Technology Solutions to discover advanced solutions customized to meet your AI requirements.